At this year’s Drucker Forum, an eye-opening discussion led by Santiago Iniguez, David Weinberger and André Reichow-Prehn raised a pivotal question: Will AI change the way we think? The debate sparked some profound reflections- not only about the potential opportunities and limitations of technology, but also about what it truly means to reason...
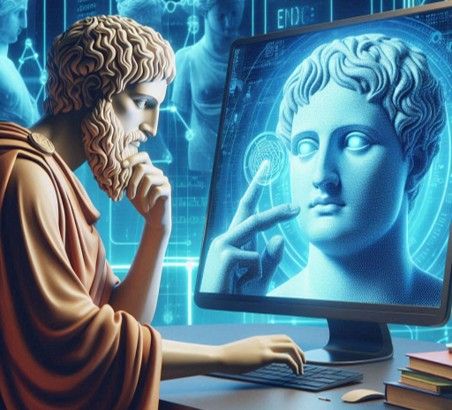
The World of AI Is Flat
For centuries, human knowledge has been shaped by the search for universal laws, exploring causality. Our libraries are filled with textbooks that catalogue an ever-expanding array of theories and models, each developed to explain natural or social phenomena and predict future events or anticipate the behaviour of human interventions. These works draw on centuries of scientific progress, from Newtonian physics to quantum mechanics, providing frameworks that help us understand everything from the motion of celestial bodies to the interactions of subatomic particles. The cornerstone of our quest for understanding is the “hypothetico-deductive” model of scientific inquiry: we observe regularities in the world, propose "covering laws" or generalizations, and test these through experimentation, empirical validation, and falsification.
But that’s not how Generative AI works. Unlike scientific inquiry, GenAI doesn’t attempt to understand causality. Instead, it operates within a flat ontology, where complex events are simplified into patterns of association. It processes data as aggregates of correlations, not causations - lacking depth, context, and intentionality. This has significant consequences:
- No Understanding of Generative Mechanisms: AI doesn’t engage with the underlying structures that generate observable phenomena. It identifies patterns but lacks the capability to understand the causative factors behind those patterns.
- The Absence of Absences: AI fails to recognize the significance of what is missing or unconscious in its data, overlooking elements that may be essential to the full context.
Epistemic Relativism
This ontological flattening also means that AI’s representation of reality is confined to the empirical level, reduced to a vast array of particulars. Words and concepts are placed like leaves on a tree, with proximity between them expressed as probabilities. In this model, everything is potentially connected to everything else. This has profound implications for AI’s capabilities:
- Deep Analysis of Patterns: AI excels in detecting patterns across vast datasets, uncovering potential correlations between data points that may not be immediately apparent. This ability is key in fields such as predictive analytics, where AI can forecast trends by recognizing hidden relationships in the data.
- "Illusions of Truth": While AI can generate outputs that seem coherent and convincing, results may lack true logical grounding. An example of this is the case where AI mistakenly relied on irrelevant visual impurities on medical scans to "predict" diseases.
However, it also means AI is incapable of “explanatory critique”, assessing internal contradictions, cultural biases, or the socio-historical evolution embedded in its data. As André Reichow-Prehn observes, had AI been developed 1,000 years ago, it would most probably still assert that the sun revolves around the Earth. This highlights how AI is shaped entirely by its training data, potentially amplifying existing biases and blind spots. By treating all input data as equally valid, it might unquestioningly aggregate conclusions across incommensurable worldviews, treat fake information as genuine, or misinterpret historical contingencies. This could also expose AI to systematic manipulation, as seen in the illegitimate distortion of scientific data by the tobacco industry around smoking and cancer. Without critical engagement, AI risks becoming a victim of epistemological relativism, producing analyses that further obscure or distort underlying realities.
1. The World of AI Is Flat
For centuries, human knowledge has been shaped by the search for universal laws, explaining causality. Our libraries are filled with textbooks that catalogue an ever-expanding array of theories and models, each developed to explain natural or social phenomena and predict future events or anticipate the behaviour of human interventions. These works draw on centuries of scientific progress, from Newtonian physics to quantum mechanics, providing frameworks that help us understand everything from the motion of celestial bodies to the interactions of subatomic particles. The cornerstone of our quest for understanding is the “hypothetico-deductive” model of scientific inquiry: we observe regularities in the world, propose "covering laws" or generalizations, and test these through experimentation, empirical validation, and falsification.
That’s not how Generative AI works. Unlike scientific inquiry, GenAI doesn’t attempt to understand causality. Instead, it operates within a flat ontology, where complex events are simplified into patterns of association, lacking depth, context, and intentionality. It processes data as aggregates of correlations, not causations. This has significant consequences:
- No Understanding of Generative Mechanisms: AI doesn’t engage with the underlying structures that generate observable phenomena. It identifies patterns but lacks the capability to understand the causative factors behind those patterns.
- The Absence of Absence: AI fails to recognize the significance of what is missing or unconscious in its data, overlooking elements that may be essential to the full context.
2. Epistemic Relativism
This ontological flattening also means that AI’s representation of reality is confined to the empirical level, reduced to a vast array of particulars. Words and concepts are placed like leaves on a tree, with proximity between them expressed as probabilities. In this model, everything is potentially connected to everything. This has profound implications for AI’s capabilities:
- Deep Analysis of Patterns: AI excels in detecting patterns across vast datasets, uncovering potential correlations between data points that may not be immediately apparent. This ability is key in fields such as predictive analytics, where AI can forecast trends by recognizing hidden relationships in the data.
- "Illusions of Truth": While AI can generate outputs that seem coherent and convincing, results may lack true logical grounding. An example of this is the case where AI mistakenly relied on irrelevant visual impurities on medical scans to "predict" diseases.
However, it also means AI is incapable of “explanatory critique”, assessing internal contradictions, cultural biases, or the socio-historical evolution embedded in its data. As André Reichow-Prehn observes, had AI been developed 1,000 years ago, it would most probably still assert that the sun revolves around the Earth. This highlights how AI is shaped entirely by its training data, potentially amplifying existing biases and blind spots. By treating all input data as equally valid, it might unquestioningly aggregate conclusions across incommensurable worldviews, treat fake information as genuine, or misinterpret historical contingencies. This could also expose AI to systematic manipulation, as seen in the illegitimate distortion of scientific data by the tobacco industry around smoking and cancer. Without critical engagement, AI risks becoming a tool for epistemological relativism, producing analyses that further obscure or distort underlying realities.
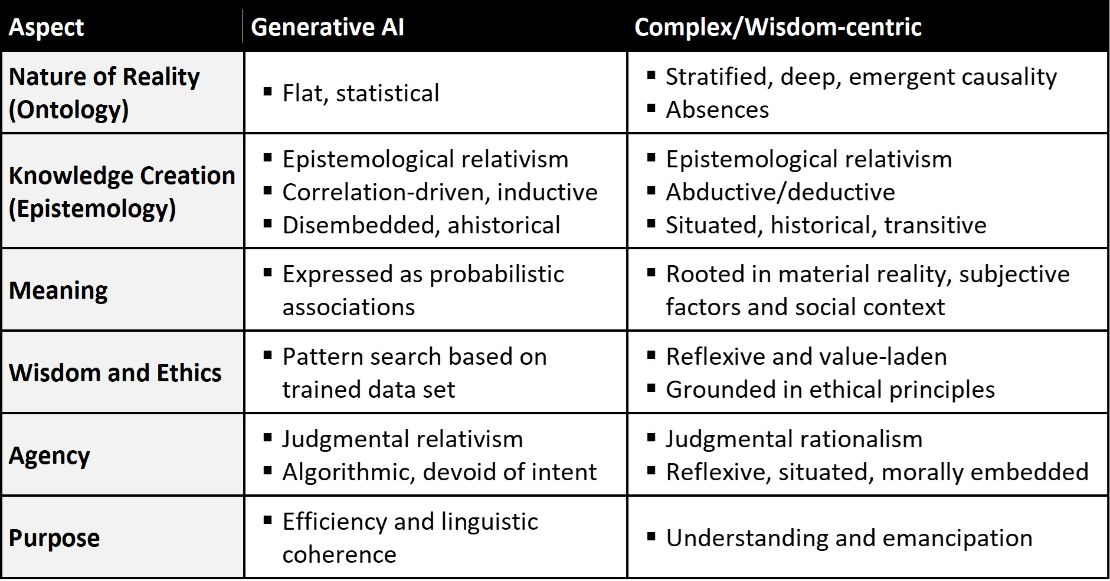
(Table: Illustrative - based on Critical Realism as Social Ontology)
Ethical Blindness
This brings us to the ethical challenge. Like science, classical philosophy has often sought to identify universal ethical principles. Take Plato's Republic, for instance, where Socrates engages in dialectical discussion with interlocutors like Thrasymachus and Glaucon to define the universal virtue of justice. Through rigorous critical reasoning, Socrates deconstructs the flawed assumptions and contradictions in Thrasymachus' claim that justice is merely "the advantage of the stronger." Then, addressing Glaucon’s famous challenge that no one would desire justice as a universal virtue, the dialogue deepens into a more profound understanding of justice—not just as an individual virtue but as an emergent institutional quality that arises when virtuous individuals fulfill their roles within a harmonious social order. In this view, justice cannot be reduced to individual gain or power; instead, it is intricately tied to rightful relationships between citizens in the realisation of the telos of shared human flourishing. In the Socratic dialogues, moral knowledge arises from active participation, critical questioning, and iterative refinement of ideas.
By contrast, generative AI approaches the question of justice through probabilistic statistical methods based on vast datasets. When asked "What is the virtue of justice?" a natural language processing model like ChatGPT tokenizes the input and encodes it into vectors that represent the statistical relationships between words. Through self-attention mechanisms, the model identifies patterns in how similar phrases appear across its training data. Each token is contextualized by weighing its relevance to all others in the input sequence, creating a hierarchical context. The outputs pass through a series of non-linear transformations, adjusting token relationships based on patterns learned during training. In the final layer, the model predicts the next word by generating a probability distribution over its vocabulary, calculated using a softmax function, where each word is assigned a likelihood score. For example, after processing the phrase "What is the virtue of," the model might assign a 45% probability to "justice," 25% to "fairness," and negligible probabilities to unrelated terms. The actual word is selected using techniques like top-k sampling (to limit choices to the most likely options) or temperature scaling (to control randomness). Thus, AI assembles sentences word by word, relying on correlations in its training data, which could include contradictory philosophical texts, summaries, and interpretations.
Most critically, the "knowledge" generated by AI is a synthesis of statistical correlations derived from its training data. While its outputs may be syntactically correct, they are semantically hollow—the system prioritizes optimization for efficiency and linguistic coherence; symbols are processed without any understanding of their meaning or connection to a deeper moral or teleological purpose.
Wisdom and Judgment: The Human Edge
Now, we can begin to appreciate the fundamental challenges of integrating AI into human decision-making. Making sound choices under uncertainty demands wisdom—the ability to apply knowledge effectively in specific contexts. Aristotle’s concept of practical wisdom (phronesis) illustrates this well: it involves a “syllogism” where universal ethical principles (major premises) are applied to particular situations (minor premises) through deliberate, context-sensitive action.
We have already noticed that Generative AI lacks the ability to comprehend ethical principles as universal foundations (the major premise). Its reliance on statistical correlations fosters not only epistemological but also ethical relativism. Additionally, AI faces stark limitations with regard to the minor premise. As a closed system, it cannot discern the salient features of a given situation or engage with diverse stakeholders to explore a range of perspectives. Most importantly, AI lacks self-reflexivity—the ability to perceive itself as part of a broader, interdependent system. It cannot discern the course of action that best aligns with universal principles while also realizing its unique role in bringing those principles to life within the specific context and affordances at hand.
This might be readily illustrated by considering a typical court case. To deliver a just verdict, a judge or jury must evaluate the facts, including the relevance of missing, unreliable, or incomplete evidence, while reconciling potentially conflicting witness statements. They must also consider the specific context and human experiences involved, such as the underlying motives of the plaintiff and defendant, along with psychological or emotional factors. Finally, they must adapt the rules of law and potential sanctions to the unique circumstances of the case, evaluating what action would have been most appropriate within the context.
All of this renders the act of judging profoundly human, requiring not only the ability to "read between the lines" and assess the weight and context of each piece of evidence but also the exercise of empathy, intuition, and moral judgment—qualities that AI cannot replicate. AI cannot distinguish between lies, lapses of memory, or differing interpretations of facts stemming from varying vantage points or worldviews. Moreover, it lacks the ability to grasp the nuances of human emotion, the subtleties inherent in language, or the diverse cultural contexts in which testimonies are given. Not even to mention any potential biases inherent in the selection of available training data.
When Data Becomes Dogma: Quo Vadis, AI?
So, will AI change the way we think? It likely already has, but I reckon the question we should rather ask is whether AI should change the way we think. As the old adage goes, "a little knowledge is a dangerous thing," and the same is certainly true for Generative AI.
(Table: Illustrative)
3. Ethical Blindness
This brings us to the ethical challenge. Like science, classical philosophy has often sought to identify universal ethical principles. Take Plato's Republic, for instance, where Socrates engages in dialectical discussion with interlocutors like Thrasymachus and Glaucon to define the universal virtue of justice. Through rigorous critical reasoning, Socrates deconstructs the flawed assumptions and contradictions in Thrasymachus' claim that justice is merely "the advantage of the stronger." Then, addressing Glaucon’s famous challenge that no one would desire justice as a universal virtue, the dialogue deepens into a more profound understanding of justice—not just as an individual virtue but as an emergent institutional quality that arises when virtuous individuals fulfill their roles within a harmonious social order. In this view, justice cannot be reduced to individual gain or power; instead, it is intricately tied to rightful relationships between citizens in the realisation of the telos of shared human flourishing. In the Socratic dialogues, moral knowledge arises from active participation, critical questioning, and iterative refinement of ideas.
By contrast, generative AI approaches the question of justice through probabilistic statistical methods based on vast datasets. When asked "What is the virtue of justice?" a natural language processing model like ChatGPT tokenizes the input and encodes it into vectors that represent the statistical relationships between words. Through self-attention mechanisms, the model identifies patterns in how similar phrases appear across its training data. Each token is contextualized by weighing its relevance to all others in the input sequence, creating a hierarchical context. The outputs pass through a series of non-linear transformations, adjusting token relationships based on patterns learned during training. In the final layer, the model predicts the next word by generating a probability distribution over its vocabulary, calculated using a softmax function, where each word is assigned a likelihood score. For example, after processing the phrase "What is the virtue of," the model might assign a 45% probability to "justice," 25% to "fairness," and negligible probabilities to unrelated terms. The actual word is selected using techniques like top-k sampling (to limit choices to the most likely options) or temperature scaling (to control randomness). Thus, AI assembles sentences word by word, relying on correlations in its training data, which could include contradictory philosophical texts, summaries, and interpretations.
Most critically, the "knowledge" generated by AI is a synthesis of statistical correlations derived from its training data. While its outputs may be syntactically correct, they are semantically hollow—the system prioritizes optimization for efficiency and linguistic coherence; symbols are processed without any understanding of their meaning or connection to a deeper moral or teleological purpose.
4. Wisdom and Judgment
Now, we can begin to appreciate the fundamental challenges of integrating AI into human decision-making. Making sound choices under uncertainty demands wisdom—the ability to apply knowledge effectively in specific contexts. Aristotle’s concept of practical wisdom (phronesis) illustrates this well: it involves a “syllogism” where universal ethical principles (major premises) are applied to particular situations (minor premises) through deliberate, context-sensitive action.
We have already noticed that Generative AI lacks the ability to comprehend ethical principles as universal foundations (the major premise). Its reliance on statistical correlations fosters not only epistemological but also ethical relativism. Additionally, AI faces stark limitations with regard to the minor premise. As a closed system, it cannot discern the salient features of a given situation or engage with diverse stakeholders to explore a range of perspectives. Most importantly, AI lacks self-reflexivity—the ability to perceive itself as part of a broader, interdependent system. It cannot discern the course of action that best aligns with universal principles while also realizing its unique role in bringing those principles to life within the specific context and affordances at hand.
This might be readily illustrated by considering a typical court case. To deliver a just verdict, a judge or jury must evaluate the facts, including the relevance of missing, unreliable, or incomplete evidence, while reconciling potentially conflicting witness statements. They must also consider the specific context and human experiences involved, such as the underlying motives of the plaintiff and defendant, along with psychological or emotional factors. Finally, they must adapt the rules of law and potential sanctions to the unique circumstances of the case, evaluating what action would have been most appropriate within the context.
All of this renders the act of judging profoundly human, requiring not only the ability to "read between the lines" and assess the weight and context of each piece of evidence but also the exercise of empathy, intuition, and moral judgment—qualities that AI cannot replicate. AI cannot distinguish between lies, lapses of memory, or differing interpretations of facts stemming from varying vantage points or worldviews. Moreover, it lacks the ability to grasp the nuances of human emotion, the subtleties inherent in language, or the diverse cultural contexts in which testimonies are given. Not even to mention any potential biases inherent in the selection of available training data.
When Data Becomes Dogma: Quo Vadis, AI?
So, will AI change the way we think? It likely already has, but I reckon the question we should rather ask is whether AI should change the way we think. As the old adage goes, "a little knowledge is a dangerous thing," and the same certainly is true for Generative AI.
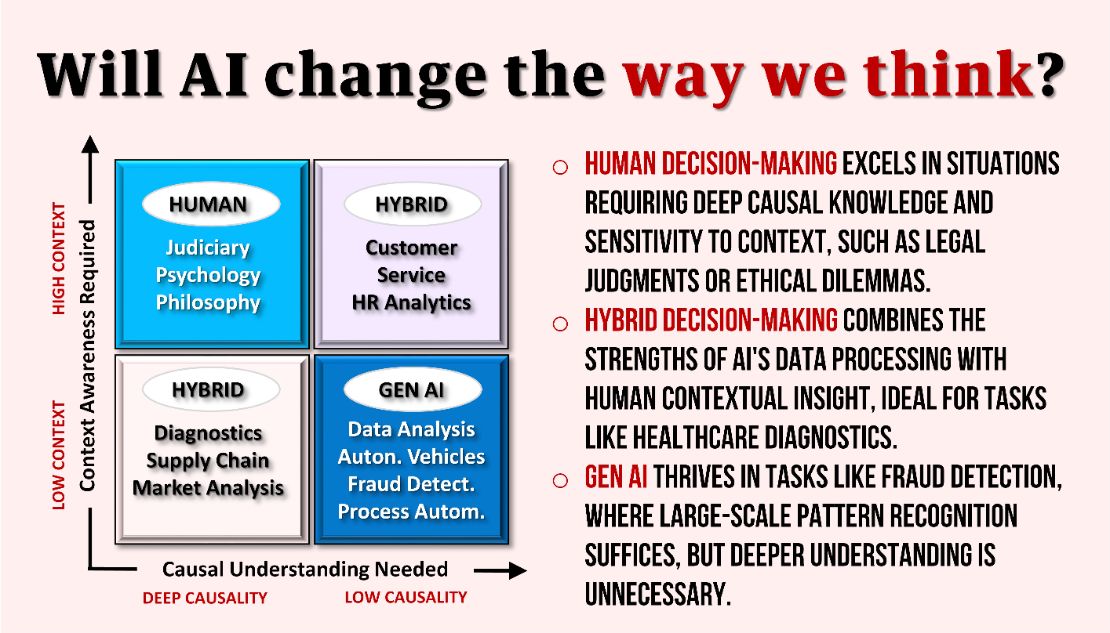
- On the one hand, there is much room for improvement in human reasoning. History shows that human judgment is often prone to error, bias, or structural flaws. In this context, AI could perhaps act as a "neutralizer," promoting a more objective application of principles or streamlining processes. For example, it could reveal discrepancies between human judgments and data-driven correlations, or assist in specific tasks such as scientific research, medical procedures and procedural automation.
- On the other hand, what humanity seems most in need of is not another algorithm to flatten our world. Rather, we require more depth in our relationships—with one another and with nature. We need empathy and moral judgment to counterbalance a focus on efficiency and instrumental thinking. It's not about speeding up email creation; it's about creating more space for reflection, to think deeply about our roles in the world. In this regard, we need “warm data”, not “big data” and further reliance on AI seems counterproductive.
So, perhaps unsurprisingly the answer is: it depends. AI should certainly be regarded as a supplementary tool for judgment. Yet, its integration into "hybrid" decision-making must be approached with caution, ensuring its limitations are fully understood. It should only be used in contexts where its deterministic and probabilistic functions are appropriate, rather than as the final authority on complex decisions. Moreover, its susceptibility to biases embedded in training data could perpetuate discriminatory practices, rather than challenge them.
I personally think the real danger lies in the risk of AI perpetually diminishing our capacity for ‘radical’ thinking, leading to some form of techno-optimistic provincialism. The power of the tools is highly seductive and we may be tempted to rely on algorithmic knowledge even when genuine wisdom is required. Thus, with AI advancing at a rapid pace, we must remain vigilant not to hollow out our own humanity, becoming masters of efficiency, but blind to the deeper meaning and purpose of our actions. Otherwise we might end up as technological giants yet spiritual dwarfs.
#genAI #wisdom #leadership #sustainability #transformation #technology
- On one hand, there is much room for improvement in human reasoning. History shows that human judgment is often prone to error, bias, or structural flaws. In this context, AI could perhaps act as a "neutralizer," promoting a more objective application of principles or streamlining processes. For example, it could reveal discrepancies between human judgments and data-driven correlations, or assist in specific tasks such as scientific research, medical procedures and procedural automation.
- On the other hand, what humanity seems most in need of is not another algorithm to flatten our world. Rather, we require more depth in our relationships—with one another and with nature. We need empathy and moral judgment to counterbalance a focus on efficiency and instrumental thinking. It's not about speeding up email creation; it's about creating more space for reflection, to think deeply about our roles in the world. In this regard, we need “warm data”, not “big data” and further reliance on AI seems counterproductive.
So, perhaps the answer unsurprisingly is: it depends. AI should certainly be regarded as a supplementary tool for judgment. Yet, its integration into "hybrid" decision-making must be approached with caution, ensuring its limitations are fully understood. It should only be used in contexts where its deterministic and probabilistic functions are appropriate, rather than as the final authority on complex decisions. Moreover, its susceptibility to biases embedded in training data could perpetuate discriminatory practices, rather than challenge them.
I think the real danger lies in the risk of AI perpetually diminishing our capacity for ‘radical’ thinking, leading to some form of techno-optimistic provincialism. The power of the tools is highly seductive and we may be tempted to rely on algorithmic knowledge even when genuine wisdom is required. Thus, with AI advancing at a rapid pace, we must remain vigilant not to hollow out our own humanity, becoming masters of efficiency, but blind to the deeper meaning and purpose of our actions. Otherwise we might end up as technological giants yet spiritual dwarfs.
#genAI #wisdom #leadership #sustainability #transformation
Popular articles in the KnowledgeHub: Critical Thinking